AI algorithms developed in the study provide a fast and tractable computational tool that, after careful validation in a clinical setting, can help tailor therapy to individual AF patients and improve their long-term wellbeing.
Principal Investigator of the study, Dr Oleg Aslanidi, Reader in Biophysical Cardiac Modelling in the School of Biomedical Engineering & Imaging Sciences
25 June 2021
AI approach could predict treatment strategy for arrhythmia patients
The proposed approach is unique in combining patient imaging, image-based modelling and artificial intelligence (AI)
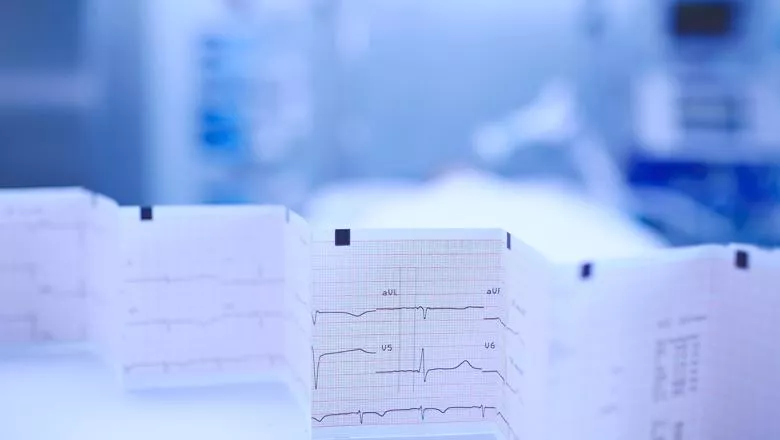
Researchers from the School of Biomedical Engineering & Imaging Sciences have proposed a novel approach to personalisation of catheter ablation therapy for atrial fibrillation (AF), a common cardiac arrhythmia that affects over one percent of the population and is associated with high levels of morbidity and mortality. The proposed approach is unique in combining patient imaging, image-based modelling and artificial intelligence (AI).
Catheter ablation is a minimally-invasive therapy where a thin tube (catheter) is inserted into the heart, and high energy passed to the catheter’s tip is used to burn areas of cardiac tissue that sustain the arrhythmia. However, ablation procedures are highly empirical, which may explain their suboptimal long-term success, with almost half of patients returning for further treatments.
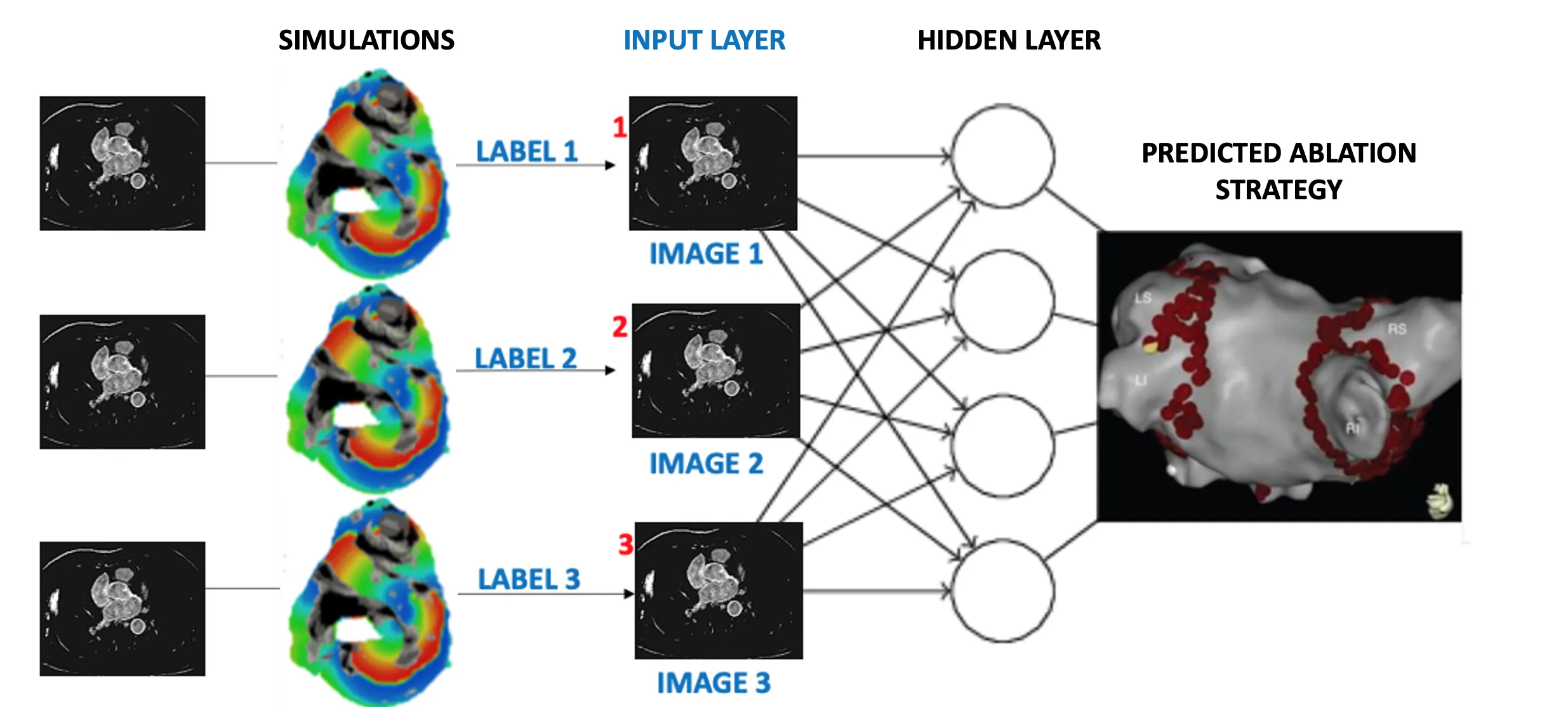
The novel approach, published in a special issue of Frontiers in Computational Physiology and Medicine, takes into account patient-specific information on structure-function relationships underlying AF, and hence AI algorithms trained on such data enable fast and accurate prediction of suitable ablation for a given patient, which can be made from clinically collected MR imaging data. Current methods only use imaging data for manual procedure guidance, missing out on the important structure-function relationships underlying arrhythmia and success of its treatment.
Currently, catheter ablation is the only treatment for arrhythmia that has a long-term curative effect. In this way it is superior to pharmacological treatments that only suppress symptoms of the arrhythmia. Existing catheter ablation strategies are highly empirical and typically target structural features in the heart that are associated with arrhythmia in all patients, without knowledge of functional changes underlying arrhythmia in each patient.
Marica Muffoletto, the first author of the study & PhD student, School of Biomedical Engineering & Imaging Sciences
Results of this study show that AI algorithms can learn from a combination of structural (imaging) and functional (modelling) patient data, to make predictions about suitable ablation strategies for a given patient. This will provide an efficient computation tool that can assist in tailoring AF ablation therapy to individual cases and improving treatment for the large patient population.