Prior to our study, only a handful of measurements derived from PET images had been found to be clinically useful for predicting response to therapy. Our paper demonstrates that “machine learning” algorithms can be trained to automatically interpret images of tumours and identify patterns in those images that are highly predictive of future clinical outcomes
Lead Author Professor Giovanni Montana, Chair in Biostatistics and Bioinformatics
14 September 2015
Artificial intelligence predicts response to cancer treatment
Researchers from King’s College London have designed an artificial intelligence system that predicts whether a cancer patient will respond to chemotherapy.
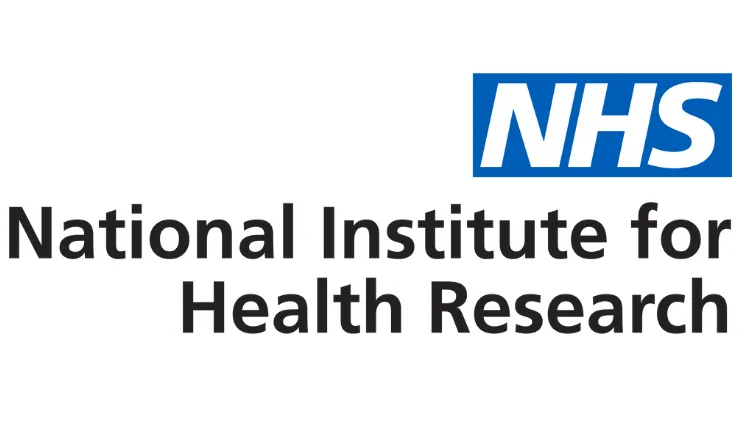
Researchers from King’s College London have designed an artificial intelligence system that predicts whether a cancer patient will respond to chemotherapy. By using a unique algorithm created using images collected from 107 patients diagnosed with oesophageal cancer, and taking a single PET image of the tumour taken before starting the therapy, the researchers have provided a way to noninvasively predict treatment response before therapy, potentially allowing oncologists to personalise future cancer treatments in the clinic.
Patients diagnosed with oesophageal cancer are often treated with chemotherapy prior to surgery. This treatment has been associated with improved survival however not all patients respond to chemotherapy. For them, the prognosis is generally even worse compared to those patients who undergo surgery only. Therefore there is a need for new technologies able to identify patients that are most likely to respond to chemotherapy as early as possible.
The researchers, from the Division of Imaging Sciences & Biomedical Engineering, used images from a PET scan which is technology able to characterize the metabolic activity of the tumour. The algorithm was designed to “understand” what features of the images were associated to the clinical outcome of interest, and eventually use those features to make predictions.
The algorithm was able to achieve a sensitivity and specificity of approximately 80%. A sensitivity of 80% means that, if we take 100 patients known to have responded to the therapy, the algorithm can correctly predict in advance that 80 of those will indeed respond. On the other hand, a specificity of 80% means that, if we take 100 patients who did not respond to the therapy, the algorithm can again correctly identify 80 of those patients, and classify them as non-responders before the therapy is started.
This research demonstrates the critical role that artificial intelligence can play towards the development of personalized cancer treatment in the clinic. Professor Vicky Goh, Chair in Clinical Cancer Imaging said, “Chemotherapy has inevitable side effects. If we know in advance that patients are unlikely to respond to chemotherapy, alternatives can be offered without delays. There are notable health care costs associated to ineffective treatment, which can be avoided in the future using these smart algorithms.”
The ability to non-invasively predict treatment response before therapy will have profound implications on the way the disease is managed, with both clinical and economic consequences. A remarkable property of the system is that it does not use any specific knowledge of oesophageal cancer and can be trained to make analogous prediction for all other cancer types.
This technique is not yet ready to be used. Professor Gary Cook, Head of Cancer Imaging, said, “We will need to further improve the performance of the algorithm in order to be considered for adoption in a clinical setting. This can only be achieved by training the algorithm on larger patient cohorts. Our findings will also need to be further validated by collecting additional data.”
First author, PhD student Petros-Pavlos Ypsilantis said, “The predictive system we developed uses artificial intelligence techniques known as 'deep artificial neural networks', which in very recent years have achieved state-of-the art performance for many different applications involving natural images; for instance, similar algorithms are currently being used by large Internet companies such as Facebook and Google for the automated recognition of faces and other objects in photographs and videos. We are currently exploring other applications in cancer and cardiac imaging".
The study was funded by the National Institute for Health Research (NIHR) comprehensive Biomedical Research Centre award to Guy's & St Thomas' NHS Foundation Trust, and King’s College London and UCL Comprehensive Cancer Imaging Centre funded by CRUK and EPSRC in association with the MRC and Department of Health.
“Predicting Response to Neoadjuvant Chemotherapy with PET Imaging Using Convolutional Neural Networks” by Petros-Pavlos Ypsilantis et al, was published on 10 September 2015 in PLOSONE .