20 July 2019
How Arthur C. Clarke's notions on AI are taking shape in the gaming industry
Dr Conor McKeown
In Arthur C. Clarke’s 1986 collection of short fiction, July 20, 2019, the various contributors make predictions about the nature of artificial intelligence in their future, now our present. Between Douglas Colligan, T.A. Heppenheimer, Clarke himself and the other contributors, it is suggested that by 2019 humanity will be served by fully functioning humanoid robots, that it will be possible to reconstruct the creative processes of artists through algorithms, there will be bases on the moon and – more outlandishly – that it will be possible to live forever by using brain transplants and experimental medication.
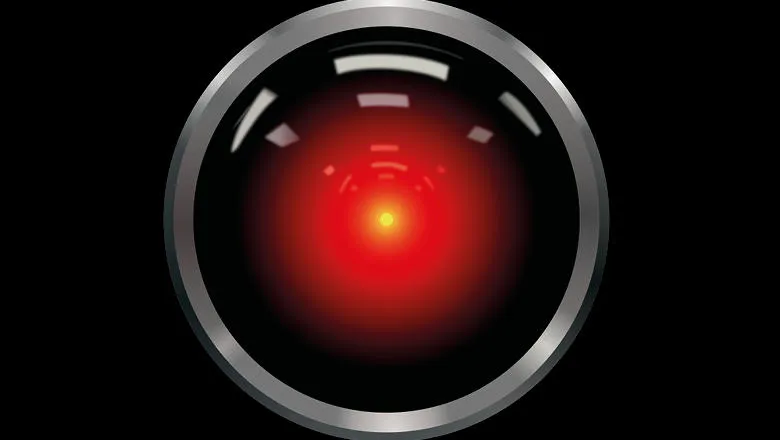
Given that the collection is a mixed bag in terms of the accuracy of the ‘predictions’, I would cautiously suggest that the value of the book really lies in providing an objective point of view on the technology of the present. It gives us a different frame of mind with which to view the technology we may take for granted today.
Reading Clarke’s collection can provide a fresh appreciation of recent technological advances. As a researcher of digital gaming, the various observations made about AI (Artificial Intelligence) in July 20, 2019, provide a refreshing point of view from which to reconsider the recent advances for AI in gaming. For example, reflecting on the predictions of fully sentient computational minds, indistinguishable from human intelligences, gives us cause to reflect on recent developments such as AlphaStar. A product of Alphabet Group’s DeepMind research group, AlphaStar is a sophisticated artificial intelligence: a series of algorithms and masses of recorded data modelled after biological systems (often called a ‘neural network’) that are refined through constant repetition.
However, rather than being programmed to replicate musical genius or fight fires, as Clarke may have predicted, AlphaStar is the next generation in a long line of artificial intelligences whose sole purpose is to play games: in AlphaStar’s case, the game it has been designed to play is StarCraft, Blizzard Entertainment’s 1998-2017’s franchise of interstellar real-time strategy games. This tradition of training computers to play games as a way of testing artificial intelligence programming began with computers trained to play games such as Tic-Tac-Toe, Chess and Go from as early as the 1950s.
However, there is one key difference that separates AlphaStar from these earlier incarnations: instead of being a product of hand-modelled rules designed by programmers to make an ultimate, for instance, Chess-playing robot, AlphaStar is instead constantly learning how to play StarCraft based on rapidly running simulations and gauging the success of potential outcomes. Don’t worry, if you’re not too sure what I’m talking about; just think of the 1983 WarGames movie. Remember how the central supercomputer, WOPR, runs millions of simulations to try and solve the problem of global war? Well, now imagine that but multiplied several times in terms of speed and complexity.
Unlike the programmed artificial intelligences of the past, designed to play games with rigid sets of rules and relatively easy to predict sequences of events, training AI to play a contemporary video game against a living human player is an almost entirely different challenge. Unlike Chess or Go, there are no best strategies in StarCraft; what’s more, there are very few ways of predicting whether actions taken early in the game have positive or negative outcomes and much of the relevant information for playing the game is initially hidden from the player.
Focusing on one small example from the game, the environment of any given level is in constant flux with new areas being uncovered through ‘scouting’. Adding to that, new objects are added to the game all the time as players create new units necessary to overcome their enemy. As such, AlphaStar is designed to constantly adapt to a changing world, coming up with new patterns based on cross referencing an enormous library of previous play simulations. As time goes on and AlphaStar runs more simulations, the better it becomes as predicting the outcome of events.
Beyond the technical differences, another quality that makes AlphaStar even more unlike AI of the past, particularly those previously designed to play StarCraft, is that AlphaStar is incredibly good at the game. So good, in fact, that is beat the human Grzegorz "MaNa" Komincz, a player of international renown, five to zero. This calls to mind previous success stories such as DeepMind’s own AlphaGo that used similar techniques to successfully win international Go championships and, of course, following in the legacy of IBM’s older (and significantly technically different in that it was not a neural network) Deep Blue. There are not many success stories in the community of game-playing AI, but when they happen, their significance cannot be overstated.
AlphaStar might seem far away from the robots and humanoid intelligences imagined by Clarke et al in July 20, 2019. It may seem a trivial thing to create a computer program (or collection of programs) to be incredibly proficient at playing a particular video game. However, we cannot take for granted the advances that have been made and how, if we could describe to Clarke what AlphaStar is capable of, just how impactful that information would be. AlphaStar represents our slow movement towards understanding one of the core properties that defines humanity and society – our intelligence. Although Clarke predicted that we would have made some more significant progress by now, being fully able to map entire personalities through computer simulations, it may be that we better understand the scale of the endeavour we have taken on. Perhaps, we have come to know just how astoundingly complex our brains are, that modelling one small ability – playing StarCraft – is such a marked achievement.
Contained in some of the many online reviews of the collection are anecdotal examples of our collective tendency to underestimate the technological sophistication of the past: reviewers frequently write of Clarke ‘predicting’ the technology of the 21st century with Nostradamus like powers. Some enthusiastic readers even write of how Clarke’s collection ‘predicted the internet’ (this wouldn’t be much of a prediction, given the internet’s inception in the 1960s and the World Wide Web’s creation in 1989/1990). However, few – if any – reviewers have written about how our understanding of the world has changed since the 1980s; how we have come to know finitude and complexity so much more keenly in our world of limited resources and inequalities. The value of Clarke’s collection, I believe, is the ability to see with fresh eyes the advances we have made – to understand AI such as AlphaStar not as trivial accomplishments but as the perception shattering robots predicted by the futurists of the past. We are slowly developing a new world and hopefully a better world; but along the way, we come to understand and appreciate just how complex our world is.