Labelling medical images at sufficient scale is very time consuming, often limiting the performance of downstream AI models. MONAI Label strives to solve this issue by providing a framework where AI models assist and accelerate clinicians in the data labelling process.
Dr Jorge M. Cardoso, Chief Technology Officer of the London Medical Imaging & AI Centre for Value Based Healthcare, Associate Professor & Senior Lecturer in AI at King's College London, and Member of the MONAI Advisory Board
20 July 2021
New MONAI tool eases the development of AI-assisted annotation models
The Medical Open Network for AI (MONAI) has released MONAI Label v0.1, an intelligent image labelling tool that speeds up the creation of annotated datasets and enables researchers to build AI annotation models easily and quickly.
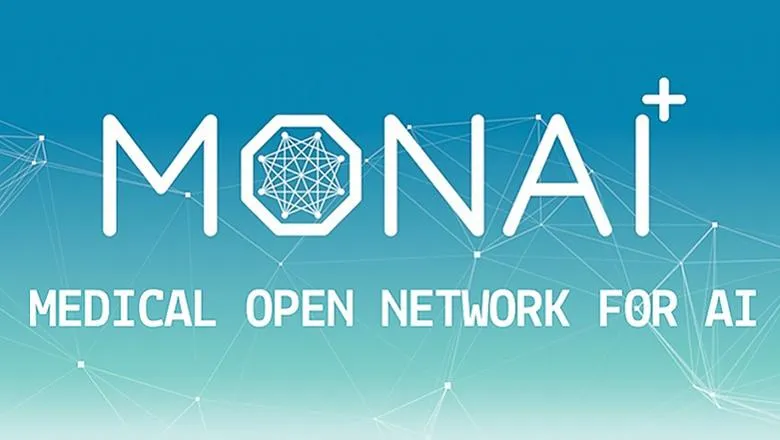
MONAI Label is an open-source system that enables the adaptation of AI models to the clinical task at hand by continuously learning from the user’s interactions and the new data label inputs.
It powers an AI-assisted annotation experience, allowing researchers and developers to make continuous improvements to their applications with iterative feedback from clinician teams, who are typically the end users of the medical imaging AI models.
Deep learning AI models require a large, curated number of labelled images to achieve good performance. Creating such annotations is tedious, time-consuming, expensive, and typically requires significant clinical expertise.
MONAI Label v0.1 addresses these issues and helps researchers and clinical teams solve medical imaging problems more efficiently. With contributions from an engaged community, the tool further aims to reduce the cost of labelling and maximise collaborations between researchers and clinicians.
The MONAI initiative was launched jointly by the London Medical Imaging & AI Centre for Value Based Healthcare, School of Biomedical Engineering & Imaging Sciences at King’s College London, and NVIDIA. It brings together AI researchers for the development and exchange of best practices for AI in healthcare imaging across academia and enterprise.