29 April 2020
Macroeconomic models in the Covid-19 crisis
Dr Francesca Monti, Deputy Director of the Qatar Centre for Global Banking & Finance
One of the crucial challenges facing epidemiologists and economists studying the evolution of the contagion and its economic effects is the lack of reliable data on the prevalence of the virus in the population.
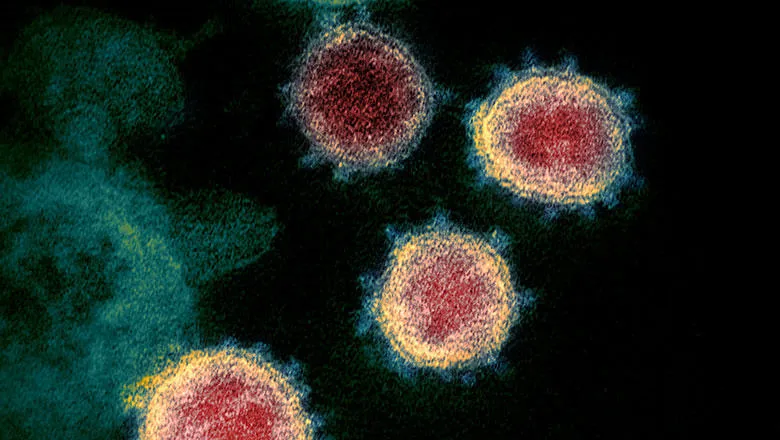
The current health crisis has triggered an economic crisis of enormous proportions. The shock, hopefully transitory, is nonetheless expected to produce the biggest fall in quarterly GDP growth in the last century at least. Attempts to forecast the evolution of the contagion, assess its economic consequences, and propose policy responses, have spurred a plethora of empirical and modelling work. The goal of this post is to zero in on a few of these contributions, highlighting the main insights and the key features of the models being developed.
Learning lessons from the past
One of the crucial challenges facing epidemiologists and economists studying the evolution of the contagion and its economic effects is the lack of reliable data on the prevalence of the virus in the population. Randomised serology testing of the population, as advocated for example by Stock (2020), would be crucial in tackling these data challenges, but the tests are still being developed and are not widely deployed as yet.
Historical data can help assess the real economic effects of a pandemic and of the subsequent public health response. For example, Jorda, Singh and Taylor (2020) use data on the rates of return on assets stretching back to the 14th century, to study the long-run economic consequences of pandemics. They find that significant macroeconomic after-effects of the pandemics persist for about 40 years, with real rates of return substantially depressed. Barro, Ursua and Weng (2020) look at data on the 1918 Flu for 43 countries and find that higher flu deaths decreased realised real returns on stocks and that flu-generated declines for GDP and consumption are on average six per cent and eight per cent.
Correia, Luck and Verner (2020) use regional data from the 1918 Flu Pandemic in the US – exploiting regional variation in severity of the pandemic and intensity on the public health interventions (e.g. social distancing measures) – and uncover two key findings. First, more exposed areas experienced a sharp and persistent decline in economic activity, and this downturn was driven by both supply-side and demand-side channels. Interestingly, however, they also find that social distancing measures do not seem to worsen the downturn: to the contrary, evidence on manufacturing activity and bank assets suggests that the economy performed better after the pandemic in areas with more aggressive public health policies. The reason is that, even if the restrictions depress economic activity when they are in place, such measures can mitigate the most severe and persistent economic disruptions, by reducing the severity of the pandemic.
To really get a sense of the channels through which these policies affect the economy, and to design appropriate policies, macroeconomic models are essential. And indeed the recent weeks have seen an explosion of newly developed models aimed at addressing the most burning economic questions of the Covid-19 crisis. We will focus on a few of these.
Integrating epidemiologic and macroeconomic models
These macroeconomic models integrate an epidemiological block to study, jointly, the dynamics of epidemics and their macroeconomic effects. Among the most common epidemiological models is the classic SIR model, proposed by Kermack and McKendrick (1927) and still used extensively, e.g. Ferguson et al (2020), to model the evolution of the contagion. The canonical model (abstracting from deaths) identifies population groups – susceptibles (S), infected (I) and recovered (R) – and describes the evolution of the disease through the whole population (N) in terms of transition equations into the various states. The discrete time version of the basic SIR model is:
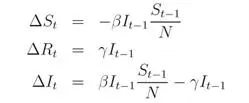
The transition probabilities between health states, β and γ, as well as the total number of people an infected person infects, R0, which is equal to the ratio of β over γ in this model, are exogenous parameters and do not account for the interaction between economic decisions and rates of infection.
Accounting for the role of economic decisions in the evolution of the pandemics
Eichenbaum, Rebelo and Trabandt (2020) address this shortcoming, by studying the interaction between economic decisions and epidemic dynamics, integrating a SIR model with an representative agent new Keynesian macroeconomic model. In their model an epidemic has both aggregate demand and aggregate supply effects. People who are working risk being infected by the virus in the workplace: they react to that risk by reducing their labour supply. The demand effect arises because the epidemic exposes people who are purchasing consumption goods to the virus and they react to that risk by reducing their consumption. The combination of supply and demand effects determine a large, persistent recession.
In this model, it is possible to prevent the infection from spreading by adopting large, permanent containment measures, proxied by a tax on consumption. Such measures however would create a persistent economic depression. Moreover, the population would never reach herd immunity and infections would recur if containment was ever relaxed. So, in this model, it is optimal to gradually ramp up containment measures as infections rise and slowly relax them as new infections wane and the population approaches the critical immunity level. This would allow to build up the fraction of the population that is immune and contain when the level of infections is high.
Understanding the uneven distribution of costs of the crisis and the policy response
The benefits and the economic costs of the pandemic and the associated mitigation policies are unevenly distributed in different sectors and groups in society, so several researchers have started looking at multi-sector models to quantify the large distributional implications of the lock-down policies that have been put in place in most countries. Glover et al. (2020), for example, build a model in which individuals can differ along several dimensions. They can be of working age or retired: the former work and are relatively less likely to face serious illness or death from infection than the latter. The latter have a higher risk of serious outcomes from the disease and are not in work, so do not bear the brunt of the economic consequences of the lock-down policies. Within the working age group, there are those working in critical sectors and those working in sectors that produce goods and services that are considered luxuries, whose jobs will be more severely affected by the lock-down policies. These differential effects imply a need for potentially large redistributive public policies. And these groups, being affected very differently by the lock-down policies, will have different views about the optimal mitigation strategy which also depend on the potential redistributive public policies put in place.
In the model by Glover et al. (2020), the household sector with heterogeneous individuals (workers in the two sectors and retired people) is integrated with an epidemiological block in which consumption, production, caring for the sick and purely social interactions affect the dynamics of the transmission, and a government block with tools to implement containment policies and redistributive policies. This model shows that the welfare gains and losses of containment policies are likely to be unevenly distributed across different segments of society. The degree of lock-down that is optimal in the model depends also on the cost of the redistributive policies, but Glover et al. (2020) suggest that, in their baseline calibration for the US, the shut-down in place on 12 April was too extensive, but that a partial shutdown in place through July would be optimal.
Liquidity matters and could significantly affect policy trade-offs
Kaplan, Moll and Violante (2020) integrate a SIR model in a macroeconomic model that can account for distributional effects and incomplete consumption insurance, as households in their model face uninsured labour income risk and health risk. In addition to the SIR epidemiological block, which is modified so that infection rates depend on economic behaviour, their richly detailed model features heterogeneity in assets (liquid and illiquid), consumption goods (regular consumption, social consumption and home production) and occupations, which are characterised by their flexibility (substitutability of work with remote work, which is less efficient) and their intensity of "social'' goods and services production.1 In this way they can account for both labour supply effects of the lock-down, coming primarily from the people who are able to work remotely, but less efficiently, and labour demand effects coming from people whose job in the non-critical goods and services industry is cut. Their preliminary findings point to the fact that the people in jobs with low flexibility, who are most affected by the containment policies, also tend to have less liquid assets and this could significantly affect the policy trade-offs.
Exactly how stark is the perceived trade-off between health and wealth?
Bodenstein, Corsetti and Guerrieri (2020) challenge the ideas that there is a trade-off between health and wealth and that social distancing policies save lives, but determine lower growth than inaction. They make this point in a two-sector growth model, which features a core sector that produces intermediate inputs not easily replaced by inputs from the other sector, subject to minimum-scale requirements. In this model, if the infection is not mitigated by social distancing measures, a much higher proportion of workers in the core sector will get ill and not be able to work and this will, because of the low substitutability of labour inputs and because of the minimum scale requirements, cause very large upfront economic costs in terms of output, consumption and investment. These results seem to be in line with the findings of Correia, Luck and Verner (2020) for the 1918 Flu Pandemic. Bodenstein, Corsetti and Guerrieri (2020) also argue that social distancing measures can reduce these costs, especially if skewed towards non-core industries and occupations with tasks that can be performed from home, helping to smooth the surge in infections among workers in the core sector.
Looking forward
The Covid-19 crisis is an epochal event that has spurred a flurry of new research and will continue to do so for some time. In this piece we focused on a few of the exponentially growing contributions on the macroeconomics of pandemics, selected because of their novel use of historical data and their focus on aspects of this crisis that are important for policy, such as the uneven distribution of the health and economic implications of the crisis. Other important issues for central banks in particular will revolve around the efficacy of monetary policy tools, the coordination of monetary and fiscal policy and the role of macroprudential policy in this crisis.
1. For example, a software engineer and an event planner have jobs that can be relatively easily done remotely, but the event planner has a much higher intensity of "social'' services production. On the other end of the flexibility spectrum, a mechanic and a waiter have jobs that cannot be done remotely, but the former produces a good that has low social intensity.
References
Barro, Robert J., José F. Ursúa, Joanna Weng, 2020. ``The Coronavirus and the Great Influenza Pandemic: Lessons from the "Spanish Flu" for the Coronavirus's Potential Effects on Mortality and Economic Activity.'' NBER Working Paper No. 26866
Bodenstein, M, Corsetti, G and Guerrieri, L. 2020. 'Social Distancing and Supply Disruptions in a Pandemic'. London, Centre for Economic Policy Research. https://cepr.org/active/publications/discussion_papers/dp.php?dpno=14629
Correia, Sergio and Luck, Stephan and Verner, Emil, Pandemics Depress the Economy, Public Health Interventions Do Not: Evidence from the 1918 Flu (March 30, 2020). Available at SSRN: https://ssrn.com/abstract=3561560 or http://dx.doi.org/10.2139/ssrn.3561560
Eichenbaum, Martin S., Sergio Rebelo, and Mathias Trabandt, 2020. ``The Macroeconomics of Epidemics.'' NBER Working Paper No. 26882
Ferguson, Neil M., Daniel Laydon, Gemma Nedjati-Gilani, Natsuko Imai, Kylie Ainslie, Marc Baguelin, Sangeeta Bhatia, Adhiratha Boonyasiri, Zulma Cucunubá, Gina Cuomo-Dannenburg, Amy Dighe, Ilaria Dorigatti, Han Fu, Katy Gaythorpe, Will Green, Arran Hamlet, Wes Hinsley, Lucy C Okell, Sabine van Elsland, Hayley Thompson, Robert Verity, Erik Volz, Haowei Wang, Yuanrong Wang, Patrick GT Walker, Caroline Walters, Peter Winskill, Charles Whittaker, Christl A Donnelly, Steven Riley, Azra C Ghani, 2020. “Impact of non-pharmaceutical interventions (NPIs) to reduce COVID19 mortality and healthcare demand.” https://www.imperial.ac.uk/media/imperial-college/medicine/sph/ide/gida-fellowships/Imperial-College-COVID19-NPI-modelling-16-03-2020.pdf
Glover, Andrew, Jonathan Heathcote, Dirk Krueger and Jose-Victor Rios-Rull, 2020. ``Health versus Wealth: On the Distributional Effects of Controlling a Pandemic.'' Mimeo. http://www.jonathanheathcote.com/healthwealth.pdf
Jordà, Òscar, Sanjay R. Singh, Alan M. Taylor. 2020. “Longer-Run Economic Consequences of Pandemics,” Federal Reserve Bank of San Francisco Working Paper 2020-09. https://doi.org/10.24148/wp2020-09
Kaplan, Greg, Benjamin Moll and Gionluca Violante, 2020. "Pandemics According to HANK.'' Mimeo.
Kermack, William Ogilvy, and Anderson G. McKendrick A Contribution to the Mathematical Theory of Epidemics,î Proceedings of the Royal Society of London, series A 115, no. 772 (1927): 700-721.
Stock, James H., 2020. Coronavirus Data Gaps and the Policy Response to the Novel Coronavirus