The purpose of this review was to identify where we can make improvements, so that we have the most robust, reliable artificial intelligence going forward towards clinical implementation. Ultimately, we want to provide safe technology that patients can really benefit from.
Dr Robert O'Shea
06 May 2021
Review identifies guidelines for better design of AI publications
The researchers identify improvements that can be made, so that we have the most robust, reliable artificial intelligence going forward towards clinical implementation
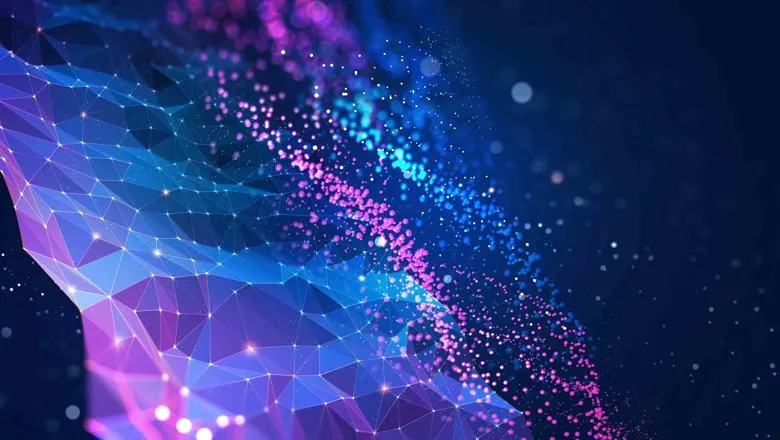
Researchers from the School of Biomedical Engineering & Imaging Sciences performed a systematic review of design and reporting of Artificial Intelligence (AI) models for radiological cancer diagnosis, providing a contemporary evaluation of quality, transparency, and reproducibility in the field. The research published in European Radiology, identified data description and model evaluation as key areas for improvement.
In recent years, researchers from the distinct fields of AI and radiology have begun collaborating to improve clinical decision-making with data science.
However, clinicians and data scientists have distinct criteria for what makes a good study. Clinicians focus on the biological and practical value of the project, whilst AI researchers examine the models and statistical aspects of the work.
Clinical AI research must stand up to scrutiny from both sides, leading to a lengthy list of potential standards to meet.
At the beginning of 2020, expect clinicians and AI researchers produced and consensus guideline, the Checklist for Artificial Intelligence in Medical Imaging (CLAIM), which encompasses both clinical and AI criteria.
CLAIM aims to promote clear, reproducible scientific communication about the application of AI to medical imaging, providing a framework to assure high-quality scientific reporting, however current conformity to these standards has not been formally quantified to date.
Dr Robert O'Shea, Clinical Research Fellow from the School of Biomedical Engineering & Imaging Sciences and Dr Amy Sharkey, NIHR Academic Clinical Fellow reviewed papers published in last five years, assessing current standards in AI research for cancer diagnosis according to the CLAIM guideline and key areas for improvement.
“Design and reporting guidelines are a simple way to improve the reliability of AI research in radiology, providing a checklist for researchers to use whilst planning and writing about their studies,” Dr O’Shea, said.
“This makes it easier for someone reading the paper to know that everything was done properly or to reproduce the study themselves.”
The researchers said AI models learn from experience, by identifying common patterns which can be used to make predictions.
Like human clinicians, AI models’ ability to make diagnoses improves with experience, especially when that experience is from a broad and diverse patient population.
When an experienced AI model encounters an unusual case, such as a rare disease, it is more likely to have encountered it previously. When an AI model encounters a case unlike any it has seen before, its prediction is less reliable. If the AI model has only seen a small group of patients from a single hospital, it may not perform well in diverse populations from different hospitals.
For this reason, researchers must describe the patient population that they have used to develop their AI model and this is one of the priorities for improvement identified in the review.
“A related issue is that of AI model testing and evaluation. To get an idea of how reliable an AI model’s diagnoses are, we need to check how it performs on cases which it hasn’t seen before,” Dr O’Shea said.
“We do this by developing the model using a proportion of cases, setting the rest aside for testing. Ideally, we use cases from a different hospital to evaluate the model’s predictions, this way we get an idea of how it will perform outside of the institution at which it was developed.”
Dr O’Shea said this “external” testing takes the AI model outside of its comfort zone to see how it rises to the task in the outside world.
However, it requires additional collaboration and data-sharing between institutions, so it is not always an easy stage of the project. This is another key area for improvement identified by the researchers’ project.
The review found that standards improved over the course of the five years examined.
Dr O’Shea expects that with the publication of the CLAIM guidelines and this review, that standards will continue to improve.
“Many of the items in the CLAIM checklist add minimal additional burden to researchers, requiring only additional documentation. In fact, adoption of CLAIM guidance may streamline projects through improved organization and anticipation of potential problems, he said.
The consensus guidelines have only been published quite recently and so we will be excited to see if standards improve over the course of the next couple of years and it will be quite nice to do a follow-up and see the establishment of these guidelines.
Dr Robert O'Shea