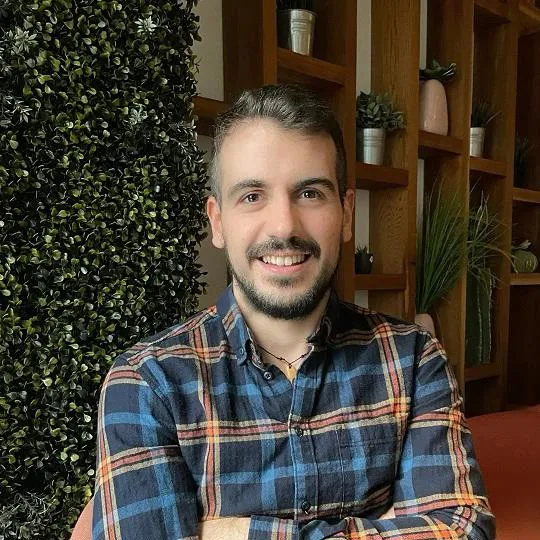
Dr Sergio Mena Ortega PhD
Research Assistant in Translational Multimodal Data Science
Biography
Sergio graduated with first-class honours in Biomedical Engineering from the Polytechnical University of Valencia, Spain. He then pursued an MSc in Biomedical Engineering: Medical Physics and Imaging at the Department of Bioengineering, Imperial College London, where he graduated with first-class honours in 2020. Subsequently, Sergio completed a PhD in Biomedical Research within the same department at Imperial College London.
Under the supervision of Dr. Parastoo Hashemi, Sergio's PhD thesis focused on the development of computational and analytical platforms for the study of neurochemical data. Specifically, he developed machine learning methods for predicting serotonin concentrations in vivo and mathematical models of the pharmacodynamic effects of antidepressants on the serotonin system.
In the AIM lab, Sergio's focus is developing machine learning models trained with multimodal data (clinical, environmental, neuroimaging, etc.) for the early diagnosis of psychosis and depression, and providing these models to the broader scientific community.
Research Interests
- Machine learning for precision psychiatry
- Psychosis and depression
- Neuroimaging
Key Publications
Mena, S., Dietsch, S., Berger, S. N., Witt, C. E., & Hashemi, P. (2021). Novel, User-Friendly Experimental and Analysis Strategies for Fast Voltammetry: 1. The Analysis Kid for FSCV. ACS Measurement Science Au, 1(1), 11–19. https://doi.org/10.1021/acsmeasuresciau.1c00003
Hersey, M., Reneaux, M., Berger, S. N., Mena, S., Buchanan, A. M., Ou, Y., Tavakoli, N., Reagan, L. P., Clopath, C., & Hashemi, P. (2022). A tale of two transmitters: serotonin and histamine as in vivo biomarkers of chronic stress in mice. Journal of Neuroinflammation, 19(1), 167. https://doi.org/10.1186/s12974-022-02508-9
Hersey, M., Samaranayake, S., Berger, S. N., Tavakoli, N., Mena, S., Nijhout, H. F., Reed, M. C., Best, J., Blakely, R. D., Reagan, L. P., & Hashemi, P. (2021). Inflammation-Induced Histamine Impairs the Capacity of Escitalopram to Increase Hippocampal Extracellular Serotonin. The Journal of Neuroscience, 41(30), 6564–6577. https://doi.org/10.1523/jneurosci.2618-20.2021
Witt, C. E., Mena, S., Honan, L. E., Batey, L., Salem, V., Ou, Y., & Hashemi, P. (2022). Low-Frequency Oscillations of In Vivo Ambient Extracellular Brain Serotonin. Cells, 11(10), 1719.
Research
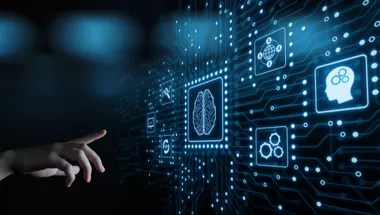
Artificial Intelligence in Mental Health (AIM)
The Artificial Intelligence in Mental Health (AIM) lab is co-led by the Chair of Precision Psychiatry Professor Nikolaos Koutsouleris and the Lecturer in Artificial Intelligence in Mental Health Dr Paris Alexandros Lalousis. It is a new lab based in the Department of Psychosis Studies at the Institute of Psychiatry, Psychology & Neuroscience. Our focus is to use AI techniques to improve understanding of mental health disorders and improve diagnostic, prognostic, and treatment tools available for mental health clinicians.
Research
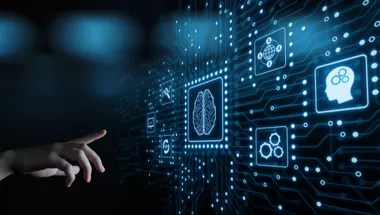
Artificial Intelligence in Mental Health (AIM)
The Artificial Intelligence in Mental Health (AIM) lab is co-led by the Chair of Precision Psychiatry Professor Nikolaos Koutsouleris and the Lecturer in Artificial Intelligence in Mental Health Dr Paris Alexandros Lalousis. It is a new lab based in the Department of Psychosis Studies at the Institute of Psychiatry, Psychology & Neuroscience. Our focus is to use AI techniques to improve understanding of mental health disorders and improve diagnostic, prognostic, and treatment tools available for mental health clinicians.