27 November 2017
Potential for machine learning to predict unknown adverse drug reactions
Lucy O'Neill
Research funded by the NIHR Maudsley Biomedical Research Centre, published in Scientific Reports today (Monday 27 November) shows that machine learning has the potential to predict unknown adverse drug reactions.
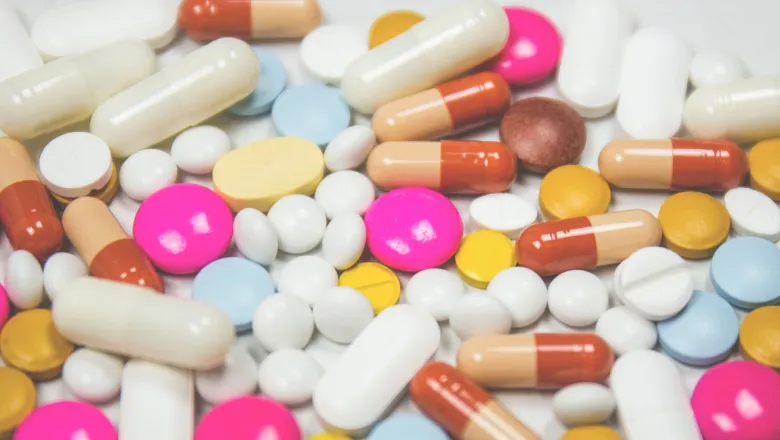
Hospital admissions resulting from adverse drug reactions (ADRs) have been projected to cost the NHS £466m. Although ADRs are monitored during clinical trials, limitations in sample size and study population mean not all ADRs of a drug will be detected before it is approved for use. Therefore only a subset of the possible adverse reactions to a drug can be discovered from clinical trials.
The King’s College London researchers developed a prediction algorithm that uses publicly available data on drugs to predict these unknown ADRs for drugs already on the market. They then used a combination of simulations and anonymised electronic health records available through the Clinical Record Interactive Search (CRIS) system developed within the NIHR Maudsley Biomedical Research Centre (BRC) to validate their predictions.
At the heart of this new method is a “knowledge graph” – a network representation of current knowledge about drugs that allows their machine learning algorithm to use the connections between different drugs to make new predictions. The research team based their algorithm on how experts reason about ADRs, allowing it to identify and ignore features that are not specific. Importantly, whilst the team initially focused on ADR prediction their method is general and can be applied to other areas such as drug repurposing, that is finding new uses for old drugs.
Dr Daniel Bean from the, IoPPN, comments, ‘Using this method, at the time a drug is marketed we can say there’s a high risk of certain additional ADRs that weren’t observed in randomized trials. That information is very valuable from a drug safety standpoint, and could even become part of the approval process for a new drug.’
To validate predictions, associations between drugs and ADRs were extracted from the free text of medical records using Natural Language Processing. Verifying predicted ADRs using electronic health records rather than relying on spontaneous reports has several advantages, the most significant being that this approach overcomes the under-reporting issues of spontaneous report databases.
However, the study authors point out that these associations alone do not prove a causal link between a drug and an ADR, and the study cannot truly establish, and report, a causal link without further manual investigation to rule out other possible causes.
The report authors were Dr Daniel Bean, Dr Honghan Wu, Professor Richard Dobson, Department of Biostatistics and Health Informatics, IoPPN, Professor Robert Stewart, Psychological Medicine and CRIS lead, Matthew Broadbent, CRIS Clinical Informatics Lead and Olubanke Dzahini, South London and Maudsley NHS Foundation Trust.
This paper represents independent research funded by the National Institute for Health Research (NIHR) Biomedical Research Centre at South London and Maudsley NHS Foundation Trust and King’s College London.
Notes to Editors
Bean, Wu, Dzahini, Broadbent, Stewart, Dobson, Scientific Reports Knowledge graph prediction of unknown adverse drug reactions and validation in electronic health records