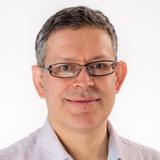
Professor Steven Gilmour
Professor of Statistics
Research interests
- Mathematics
Biography
Steven Gilmour graduated with a BSc in Statistics from Heriot-Watt University in 1987 and a PhD in Applied Statistics from the University of Reading in 1991. He was Lecturer in Applied Statistics at the University of Reading from 1990-99, Reader in Statistics in the School of Mathematical Sciences at Queen Mary University of London from 2000-04, Professor of Statistics at Queen Mary, University of London from 2004-10, and Professor of Statistics in the School of Mathematical Sciences and the Statistical Sciences Research Institute at the University of Southampton from 2010-16. He joined King's College London as Professor of Statistics in the Department of Mathematics in 2016.
Research interests
Steven Gilmour's research is mostly on the statistical theory, methodology and applications of the design and analysis of experiments. Much of his research is on experiments with complex treatment structures, such as those with many variables (factorial designs), those with continuous levels (especially leading to nonlinear models) and those with several continuous variables (response surface methodology). Problems of choice of treatments, allocation of treatments to experimental units and links between design and modelling are all of importance to experimenters and lead to interesting statistical work.
Some particular areas of recent and current research are:
-
Multi-stratum designs, in which practical restrictions on the randomisation lead to information appearing at different levels of experimental unit from different treatment factors.
-
Multi-objective optimal design in which designs are sought which meet the multiple practical requirements that experimenters usually have.
-
Designs for multifactor nonlinear models, especially hybrid models in which mechanistic information is used to describe the effects of one or more factors on the response, but purely empirical models are used for the other factors' effects.
-
Designs for connected experimental units, where the usual assumption of treatment-unit additivity is not plausible and the design and modelling have to allow for this.
-
Factorial design which are good under model uncertainty, when it is not known which effects are expected before the experiment is run.
-
Design-based modelling of data from experiments, which is driven by the randomisation carried out, as well as what is know about the effects of treatments a priori.
These methods are applicable to experiments in many fields of application, but Steve has been particularly involved with applications in healthcare, pharmaceuticals, biochemistry, food, agrochemicals, biochemical engineering, horticulture and market research.
Further information
Research
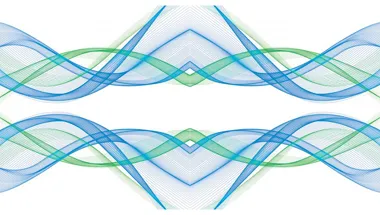
Centre for Non-Equilibrium Science (CNES)
CNES acts as an international hub for cross-disciplinary research in non-equilibrium science.
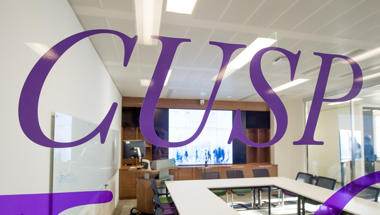
Centre for Urban Science and Progress (CUSP) London
Supporting interdisciplinary research and innovation in and for London
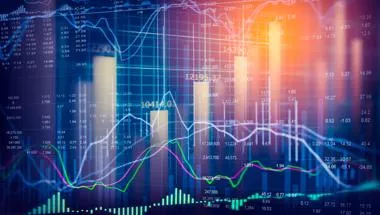
Statistics
The group has research strengths in the design and analysis of experiments, time series and Markov chain Monte Carlo and sequential Monte Carlo methods.
News
New cutting-edge Master's in Statistics launches at King's College London
The new course kicks off in September 2023 for postgraduate students with strong mathematical research backgrounds.
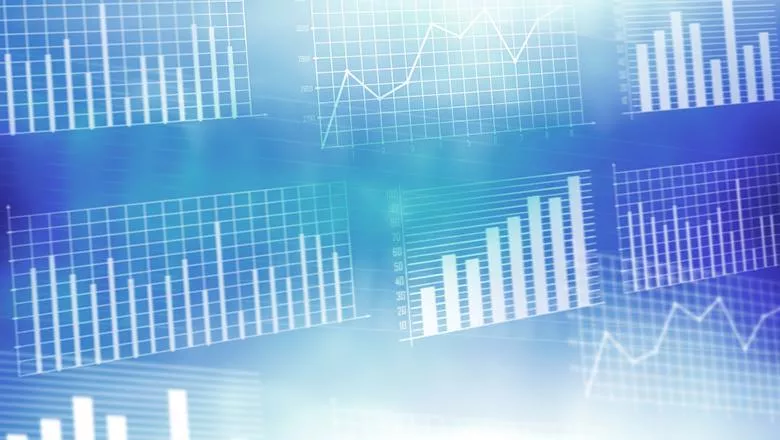
Audio-based AI unreliable for predicting Covid-19 infection, study finds
Researchers found that technology using Machine Learning performed no better than simply asking people to report their symptoms
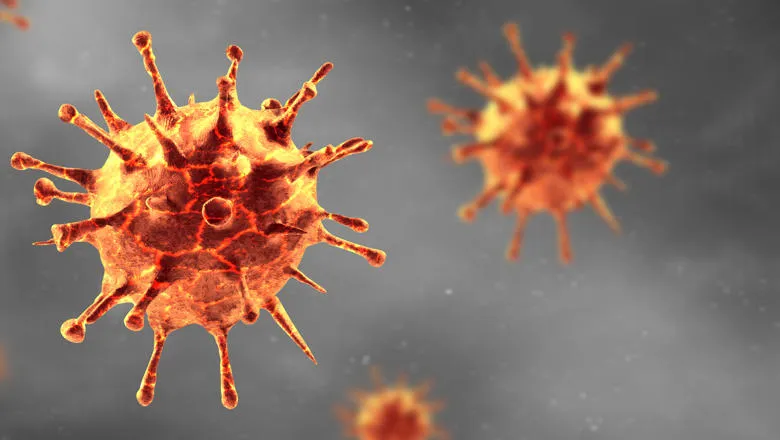
New scholarships scheme for postgraduate students
King’s is delighted to be one of the founding partners of the Martingale Foundation established to support students hoping to study for a PhD in STEM subjects.
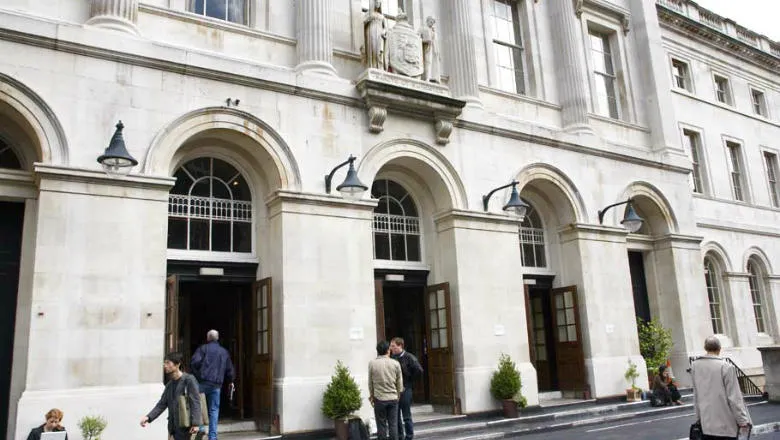
New Head of the Department of Mathematics
Professor Steven Gilmour has been appointed as the new Head of the Department of Mathematics from 1 August, taking over from Professor Reimer Kühn.
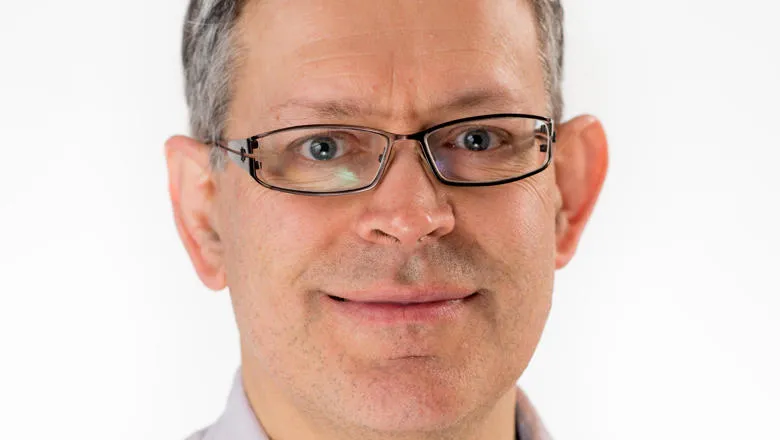
Professor Steven Gilmour wins American Society for Quality: Statistics Division's Lloyd S Nelson Award
The Department of Mathematics’ Professor Steven Gilmour, along with co-author Peter Goos from KU Leuven, Belgium, has received the American Society for...

Events

British Mathematical Colloquium 2022
The 73rd British Mathematical Colloquium, the principal cross-disciplinary meeting for pure and applicable mathematics in the UK, will take place in King's...
Please note: this event has passed.
Research
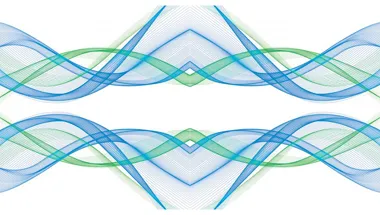
Centre for Non-Equilibrium Science (CNES)
CNES acts as an international hub for cross-disciplinary research in non-equilibrium science.
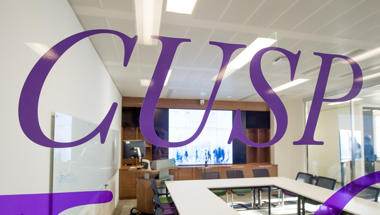
Centre for Urban Science and Progress (CUSP) London
Supporting interdisciplinary research and innovation in and for London
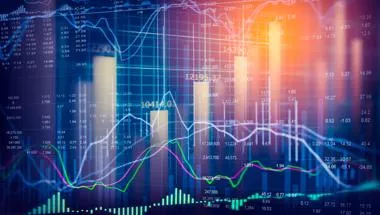
Statistics
The group has research strengths in the design and analysis of experiments, time series and Markov chain Monte Carlo and sequential Monte Carlo methods.
News
New cutting-edge Master's in Statistics launches at King's College London
The new course kicks off in September 2023 for postgraduate students with strong mathematical research backgrounds.
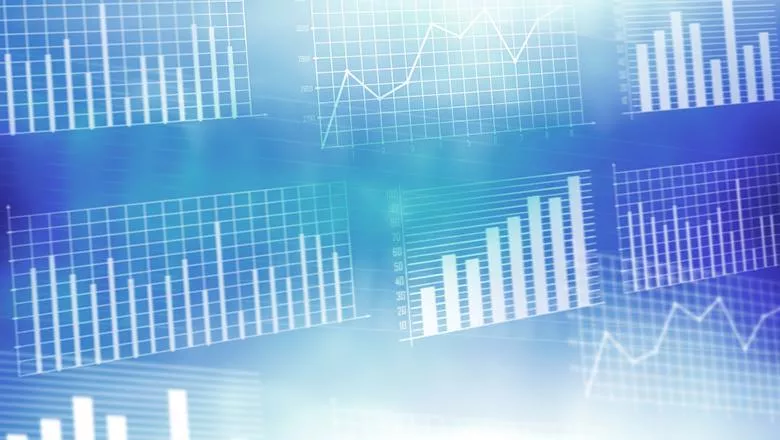
Audio-based AI unreliable for predicting Covid-19 infection, study finds
Researchers found that technology using Machine Learning performed no better than simply asking people to report their symptoms
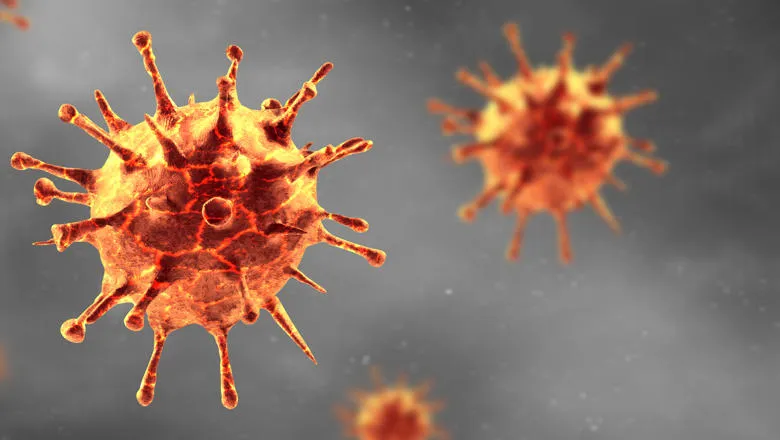
New scholarships scheme for postgraduate students
King’s is delighted to be one of the founding partners of the Martingale Foundation established to support students hoping to study for a PhD in STEM subjects.
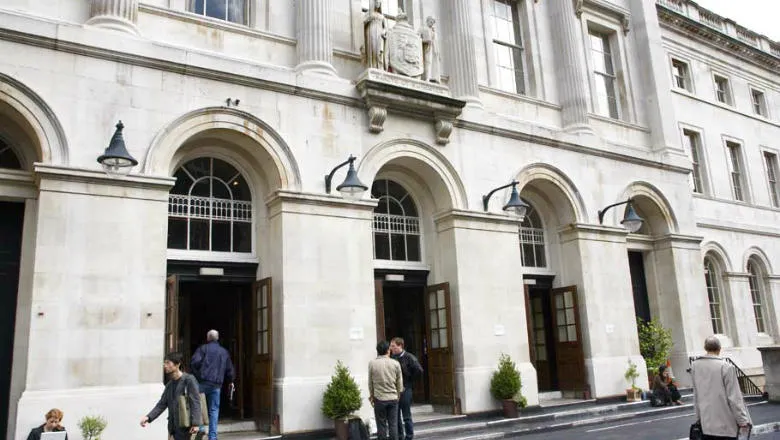
New Head of the Department of Mathematics
Professor Steven Gilmour has been appointed as the new Head of the Department of Mathematics from 1 August, taking over from Professor Reimer Kühn.
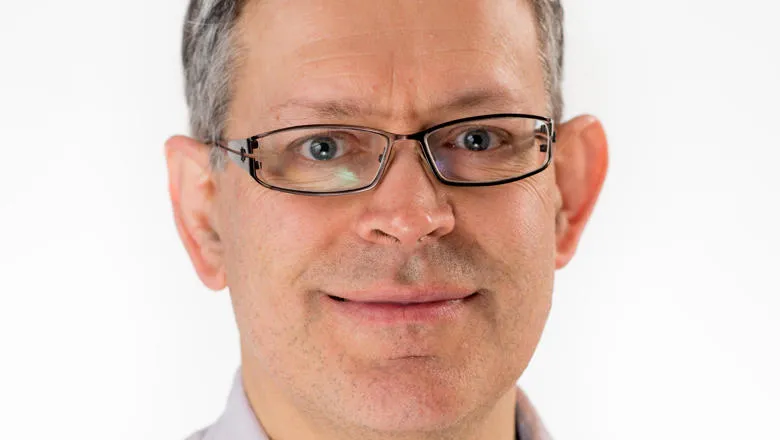
Professor Steven Gilmour wins American Society for Quality: Statistics Division's Lloyd S Nelson Award
The Department of Mathematics’ Professor Steven Gilmour, along with co-author Peter Goos from KU Leuven, Belgium, has received the American Society for...

Events

British Mathematical Colloquium 2022
The 73rd British Mathematical Colloquium, the principal cross-disciplinary meeting for pure and applicable mathematics in the UK, will take place in King's...
Please note: this event has passed.